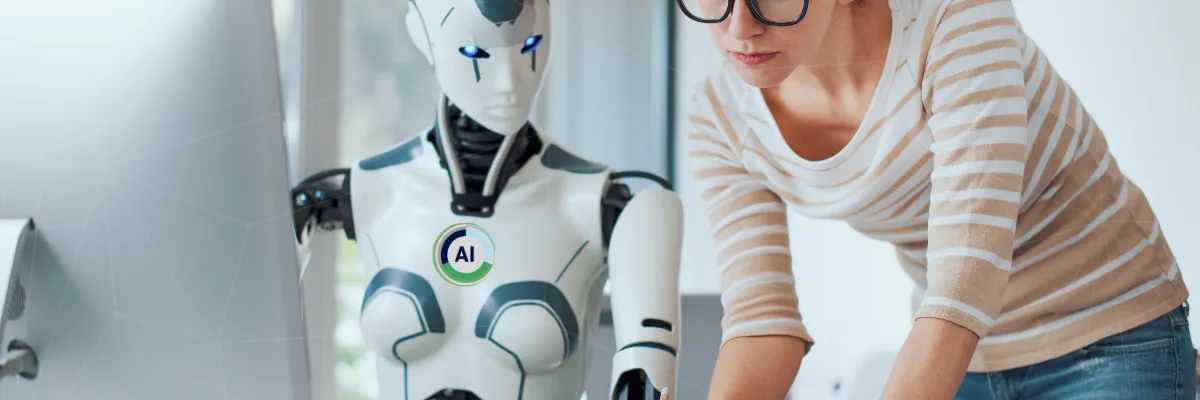
Behind the Creation of "AI Vs. Humans: The Cost of Work"
Note: This article serves as an extension of the "AI Vs. Humans: The Cost of Work" article, providing a detailed summary of how it was created. It outlines the process, tools, and research involved in bringing the topic to life. To access the original article, click here.
Blog Creation Workflow
The goal of this post was not to create a hand-crafted top-quality editorial work. The goal was to see how well we could quickly create a reasonably good overview of a topic, quickly, using top-tier AI tools currently available. Here's how we went about it:
Text Creation
This content was created using OpenAI Deep Research (using a $200/mo ChatGPT Pro account, which gives us 100 Deep Research operations per month) for the Deep Dive content and then used GPT-4o to generate the other sections. We translated some tables that didn't format well into outline format and replaced them in the original Deep Dive content and made other minor editing to improve the presentation.
Here is the initial prompt we used to start the OpenAI Deep Research into this topic:
Describe the latest research on the relative impact on using AI to do tasks/work vs the impact of having humans do the work. Include impacts on water use, carbon footprint, energy use, net and gross costs, and total net energy and environmental footprint/impact needed for similar tasks. Provide dated citations (with date, title, link, brief (1-2 sentence) summary of finding, and quality rating (1-100%, with reasons) based on study quality, peer review, etc). The goal is to have an accurate assessment of the total net costs and benefit of Human vs AI to do the work. Maintain apples-to-apples comparisons, such as if you are going to consider the cost and resources of training an AI model, also compare the cost and resources of training a Human to the point where they can do the equivalent work.
ChatGPT then requested some clarifying information:
To provide an accurate and comprehensive assessment of the total net costs and benefits of AI vs. humans for performing work, I need to clarify a few details:
Scope of Work: Are there specific types of tasks or industries you are most interested in (e.g., customer service, data analysis, software development, creative work, scientific research, manufacturing)?
Timeframe: Are you looking for research within a specific time range (e.g., past 1-2 years, past 5 years)?
Comparative Factors: You mentioned key impact areas—water use, carbon footprint, energy use, net and gross costs, and total environmental footprint. Would you like any additional metrics (e.g., labor productivity, economic output, ethical considerations)?
Study Quality Threshold: Do you have a minimum threshold for study quality (e.g., peer-reviewed journals only, government/NGO reports, reputable industry analyses)?
Regional Focus: Should this research be global, or do you want a focus on specific regions (e.g., U.S., Europe, China)?
Let me know your preferences so I can refine the research accordingly.
We responded with the following clarifications:
1. Focus on two datasets, (1) the traditional uses of AI, such as content generation, document analysis, code generation, etc (search for most common use cases) and (2) deeply technical uses such as Deep Mind, Alpha Folding, and recent Google Co-Scientist work. 2. For AI applications within the last 3 years (the GenAI Age) 3. Other factors such as Dollars-Generated vs Dollars-Cost, or other metrics focused on the sustainability of AI as a work product creator. 4. No minimal threshold, but estimate the quality and weight accordingly. 5. US, UK, EU primarily. Rest of the world as a secondary focus.
It then got to work, with the following reply:
Understood. I will research the latest studies from the past three years (the GenAI era) comparing AI-driven work to human work, focusing on two primary datasets:
Traditional AI applications (e.g., content generation, document analysis, code generation)
Deep technical AI applications (e.g., DeepMind’s work, AlphaFold, Google’s Co-Scientist project)
The research will include analyses of:
Water use
Carbon footprint
Energy use
Gross and net costs
Total environmental impact
Economic factors (e.g., Dollars-Generated vs. Dollars-Cost, sustainability of AI work product)
I will prioritize sources from the US, UK, and EU, with secondary focus on global perspectives. Each citation will include date, title, link, summary, and a quality rating based on peer review, methodology, and relevance.
I’ll update you once I have gathered the findings.
Research completed ten minutes later, after consulting 36 sources
Research completed in 10m · 36 sources
Here is the reasoning steps it documented along the way:
AI vs. Human Work: Energy, Cost, and Environmental Impact
I’m comparing AI-driven work to human work in terms of water, carbon footprint, energy consumption, costs, environmental impact, and economic sustainability. This involves examining the resource investment in training AI models versus training humans. Herausfinden clearly affects efficiency.
Specifically, I’m identifying credible studies and reputable sources to compare AI and human work, focusing on US, UK, and EU research, and prioritizing peer-reviewed studies for reliability and quality.
Searched for AI water usage in 2022
I’m thinking through various sources like MIT News and ArXiv. ArXiv’s paper on AI models' water footprint seems particularly relevant.
Read arxiv.org
I’m piecing together the water footprint of AI models, considering training impacts and generative tasks. Fascinated by the specific water use details for these models.
Read more from arxiv.org
I'm weighing the likelihood that ArXiv houses the necessary details.
Read more from arxiv.org
I am assessing the water usage of GPT-3 and investigating its carbon footprint, energy consumption, and economic sustainability.
I'll explore the "Co-Scientist AI" term further to understand its context and application.
Searched for Co-Scientist term
I'm pulling together information about Google's "AI co-scientist" project, likely emerging in late 2023, as part of their multi-agent system innovations.
Read reuters.com
I’m noting Alphafold's training energy costs to explore environmental and cost implications. This approach helps gauge its broader impact.
Searched for AlphaFold carbon footprint and energy
AlphaFold 2, released around 2021, is significant for its advanced AI capabilities, though outside the past three years. Considering new projects like AlphaCode and AlphaTensor for relevance.
Searched for AlphaCode model size and energy consumption
I'm considering an AI versus human cost ROI study to gauge AlphaCode's potential environmental impact.
Searched for AI vs human environmental cost study
I’m looking at different resources, ranging from articles and books to peer-reviewed journals. Each tackles the environmental cost of AI, spanning perspectives and methodologies.
Read news.mit.edu
I'm mapping out references from the MIT article, considering their credibility and potential inclusion of MIT news updates or statistics. This approach ensures a comprehensive analysis of the information provided.
Read more from news.mit.edu
OK, let me see: Olivetti is the senior author of a 2024 paper on climate and sustainability, likely in context with generative AI.
Read more from news.mit.edu
I'm considering the environmental impact of training and using large-scale AI models compared to human labor, focusing on energy, cost, and sustainability.
Searched for EU AI carbon footprint study 2023
OK, let me see. The snippet reveals GPT-3 175B's energy use and resulting carbon emissions.
Read news.climate.columbia.edu
Taking a closer look at the scene, I’m thinking about how movement and emotions are beautifully merged.
Read more from news.climate.columbia.edu
OK, let's think through the environmental footprint of training GPT-3. Training GPT-3 emits 502 metric tons of CO2. Daily ChatGPT use produces ~8.4 tons of CO2 per year.
Read ar5iv.org
I'm confirming that the FACETS project contributes to defining the scope and context for the NASEM study on trustworthy AI, especially considering ethical, technical, and operational factors in contractual design.
Searched for GPT-3 energy consumption 1287 MWh
OK, let me see. "Carbon Emissions and Large Neural Network Training" from April 2021 is foundational. I’m also gathering data on traditional and innovative AI tasks, focusing on energy usage and cost comparison with human tasks, and code generation.
Read kaspergroesludvigsen.medium.com
Wondering about LLaMA's carbon footprint.
Searched for LLaMA carbon footprint Meta
I’m thinking about the energy usage and carbon emissions associated with training AI models. Lingering ideas include comparing these factors with GPT-3 and considering the environmental footprint of generative art.
Searched for cost to train GPT-3 2022
I'm gathering info on the cost of AI models like GPT-3 and PaLM, and their economic impact, including potential automation benefits and hidden costs.
Read planetdetroit.org
I’m gathering info on the water usage by data centers. A 2021 study shows 7100 liters per megawatt-hour, while Google’s centers in the US consumed 12.7 billion liters in 2021.
Read more from planetdetroit.org
Planet Detroit article traces The Conversation replication, respecting authors from UTS. Information on hot water and energy usage consolidates, showing progress.
Read weforum.org
I'm gathering data on Microsoft and Google's emissions, noting the significant energy consumption of data centers. Generative AI's energy intensity, especially GPT-4, is also a major consideration.
Searched for UK AI carbon footprint study 2023 Cambridge
Taking action is key to mitigating computational science's growing carbon footprint. These efforts suggest sustainable practices are essential.
Read cam.ac.uk
I’m figuring out the energy consumption comparison between traditional AI tasks, like content creation and document analysis, and deep technical AI applications against humans. This includes evaluating upfront and ongoing costs.
Searched for AI vs human writer cost analysis
I’m looking at the financial cost and analyzing the quality of writing.
Read ediscoveryai.com
I'm piecing together the financial benefits of AI in eDiscovery, focusing on document handling efficiency and cost savings. Environmental impact and overall financial evaluation are crucial.
Read more from ediscoveryai.com
AI's efficiency becomes clear, reducing review costs to $450K from $1.705M.
Searched for AlphaFold vs X-ray crystallography environmental impact
I'm looking into how Alphafold's energy efficiency compares to X-ray crystallography, considering various setups and potential impacts.
Searched for AlphaFold training compute TPU cost
AlphaFold's heavy compute load raises questions about its overall environmental net impact. It’s worth noting that this comparison with human efforts is quite fascinating.
Read news.mit.edu
I’m gathering details on required citations: title, link, summary, and quality rating. Quality scales range from peer-reviewed to unreviewed, ensuring reliability.
Read mit-genai.pubpub.org
I'm weighing the treatise's call for sustainable development, noting its probable reliance on external sources and lack of new data.
Read more from mit-genai.pubpub.org
I'm thinking through the credibility of the sources, which include a credible internal paper with notable authors and a UK/EU commentary without a specific focus on AI humanities.
Searched for EU AI sustainability report 2023
I'm gathering info on comparative tables for AI and human progress, focusing on metrics like training time, energy, water, and costs for tasks such as content generation, document analysis, and code generation.
Read arxiv.org
Assessing AlphaFold's 11-day training cost to physical lab experiments, AlphaFold uses data centers and reduces the need for lab-based X-ray crystallography, which also incurs significant costs.
Searched for AlphaFold vs lab experiment carbon cost
I’m piecing together the environmental impacts of ML in proteins by browsing PubMed. This not only highlights ML’s potential but also its broader implications.
Considering AlphaFold's high emissions, noting its significant carbon footprint relative to vehicle use, and gathering references for comparison.
I'm comparing the economic benefits of AI-generated outputs to traditional methods, factoring in efficiency and scalability. The aim is to provide a nuanced understanding of ROI.
Searched for generative AI economic impact and ROI 2023
OK, let's think it through: AmplifAI quotes a $1 investment yielding a $3.7 return. McKinsey's June 2023 analysis estimates generative AI could add $2.6-4.4 trillion annually.
Read amplifai.com
OK, let’s see. The " (10) " most likely refers to source 10, indicating it’s numbered in their sources.
Read more from amplifai.com
I'm working through the credibility of a 3.7x ROI statement from IDC's global study. Considering a 3.5x figure might provide a more conservative estimate.
Read medium.com
I'm thinking through the environmental and economic impacts of AI and human labor over 2022-2025, comparing traditional AI tasks to human work, and assessing deep technical AI innovations.
Read pharmaphorum.com
OK, let's think this through: Alphafold's AI model boosts prediction efficiency, saving time and costs. Despite high energy needs, it’s far more effective than human expertise.
Searched for training human vs AI model
I’m thinking through the environmental and economic impacts of AI and human labor in content generation, document review, and protein folding, focusing on training energy, carbon emissions, and costs.
I'm examining AlphaFold's CO2 footprint compared to lab-based experiments, noting the vast number of structures predicted with significantly lower emissions per structure.
Evaluating references entails considering peer review status, credibility, and relevance to AI and environmental sustainability, ensuring a comprehensive evaluation of data and sources.
Read arxiv.org
ArXiv might not appear here as expected.
Read more from arxiv.org
I’m working through the quality of AI water footprint and energy research preprints, considering their credibility and progress, and noting the influence of notable authors and their findings.
Read more from arxiv.org
I’m balancing the credibility and timeline of various sources, including a Google researcher paper, MIT News, and the WEF blog, to support carbon and water mentions. Peer-reviewed style from ArXiv and WEF's referencing is considered.
Read weforum.org
I’m assessing the legitimacy of each source, noting quality ratings and relevance to environmental impact, and deciding on their inclusion in the reference list.
Read theconversation.com
The article delves into the escalating energy consumption and environmental impacts of AI models, including a detailed look at Meta's Llama, which consumed 2.6 million kWh and emitted 1000 tCO2.
Read huggingface.co
I’m compiling GPU hours, emissions, and offset details for pretraining various models, starting with Llama 2. I’ll consult sources like Arxiv, Reuters, and McKinsey to ensure data accuracy.
Searched for AI vs human document review cost
I'm cross-checking EY's findings on doc intelligence, comparing review and cost reductions with eDiscovery's analysis.
Read blog.lexcheck.com
I'm assessing references to streamline the data. Prioritizing key insights without overcrowding.
Read mckinsey.com
I'm evaluating references to include, considering credibility and relevance, such as McKinsey 2023 and WEF July 2024, to ensure a balanced and robust analysis.
Read blog.lexcheck.com
I'm working through various sources, assessing quality and considering integrating a specific IDC ROI stat.
Read blogs.microsoft.com
Ensuring report structure with headings, bullet points, and aligning references. Using bracket style for inline citations and hyperlinking for accessibility. This approach maintains clarity and engagement.
Research completed in 10m · 36 sources
We then asked it to generate the additional levels of summary with this GPT-4o prompt:
Provide a 1-2 sentence TL;DR summary, a 1-3 paragraph summary, and a bullet point outline of this content with TLDR summaries of each points
Which generated the textual content we used here.
Image Creation
For the blog post image, I then gave ChatGPT 4o the prompt:
Create 5 descriptions for a blog post title image suitable for this content and its conclusion. Then create an example image for each. Images should be 1200x400 with the key content in the center.
and it made a collection of horrible crappy glowy-icon-badtext-ai-mashup images.
I gave it another prompt:
All these look like stereotypical AI images. Can you create them with a less generic AI feel? Consider making them look more like commercial illustrations rather than space-age AI images with floating glowing things. For example, a more corporate illustration with computer vs human iconography and icons like water, energy, food, etc.
And it then gave me vector-art-looking horrible graphics.
I then took the prompt from the least horrible one of those and copied the prompt the image used:
A commercial-style infographic illustrating the environmental footprint of AI vs. human work. The image features two sections: on the left, a corporate office setting with an employee at a desk, accompanied by icons for energy use, water consumption, and carbon footprint. On the right, a stylized AI data center with icons for electricity consumption, cooling water usage, and emissions. The visual style is clean, professional, and modern, avoiding sci-fi aesthetics.
This prompt was then pasted was generated into Canva's Magic Tools (which chopped off the end due to character limits) where we made a few variations and then cropped out stuff we didn't like, added a stock image from Canva and added it to our blog header template.
We hope you have found this all interesting, and if we can help you in using AI to improve your operations, give us a shout!